Using AI, machine learning to confront biodiversity loss
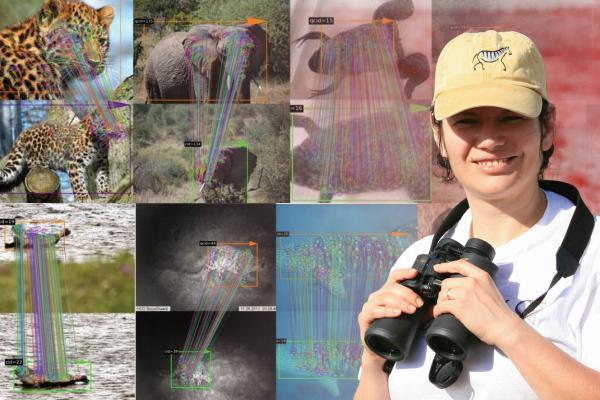
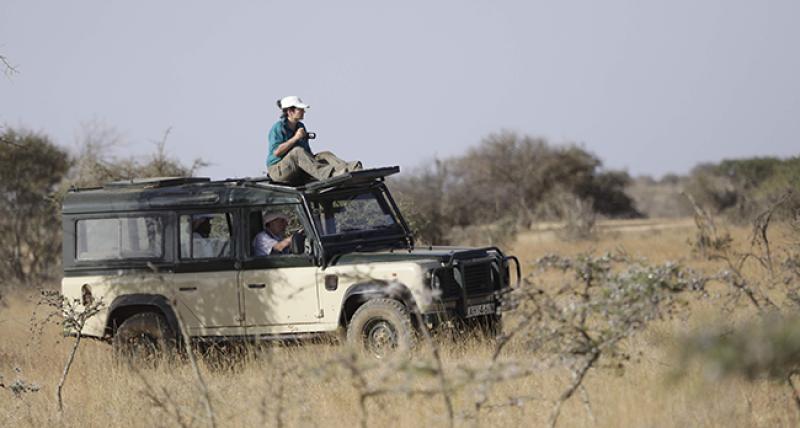
Tanya Berger-Wolf takes photos of wildlife from atop a Land Rover at Mpala Research Centre in Kenya.
Tanya Berger-Wolf was skeptical.
“You need to see your data!” insisted collaborator Dan Rubenstein, an ecologist and professor at Princeton University’s Department of Ecology and Evolutionary Biology.
Rubenstein studied zebras and wanted to learn how they interacted socially. But to do that, he needed to track the zebras and collect the resulting data. Berger-Wolf, then a computational ecologist at the University of Illinois, Chicago, parsed out that data, using it to find patterns that could reveal the animals’ networks and sociality. Rubenstein was gearing up for another field season in Kenya and was trying to convince Berger-Wolf that she needed to tag along.
But did she really? She was more comfortable cataloging that data into spreadsheets, writing algorithms and programs that could analyze and make sense of it all. What difference did it make if she actually saw the zebras up close?
But Rubenstein was adamant.
“You need to,” he said. “It will all make more sense.”
So in 2008, she relented. Berger-Wolf, Rubenstein and their colleagues arrived at the Mpala Research Centre, a wildlife conservation laboratory in Kenya's Great Rift Valley. As soon as she saw her data — the zebras, the giraffes, the elephants — dashing across the savanna, she knew Rubenstein was right.
“I completely fell in love,” she said. “It was amazing.”
Fourteen years later, Berger-Wolf, now a professor of evolution, ecology and organismal biology at Ohio State, looks back on that first trip to Kenya as a watershed moment in more ways than one. It led to a groundbreaking project that used artificial intelligence to identify and track individual zebras via their stripes. It inspired the creation of Berger-Wolf’s nonprofit, Wild Me, which uses machine learning to build open-source software for conservationists. It breathed life into an entirely new field of study, imageomics, which through the upcoming Imageomics Institute at Ohio State will allow scientists to extract biological data from a massive cache of digital images. For Berger-Wolf, it led her to a fascinating new intersection she can call her own, one where AI and ecology converge, one where she is free to discover, experiment and innovate.
It sounds corny, but I’ll say it anyway: I’m doing exactly what I want to be doing,” said Berger-Wolf, also a professor in the College of Engineering. “This privilege of thinking, ‘Oh, I’d like to do this,’ and then going ahead and doing it is incredible.”
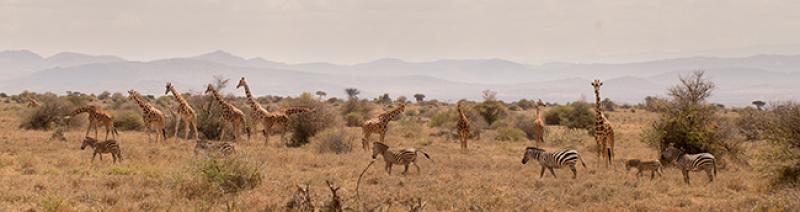
Meeting the Next Mass Extinction
Humanity was the worst thing to happen to just about every other living creature on Earth.
The human impact on the environment — things like deforestation, anthropogenic climate change, habitat destruction, ocean acidification — is causing the largest biodiversity loss in human history. Earth’s “normal” extinction rate — the rate at which species naturally die off — is thought to be around 0.1 to 1 species per 10,000 every 100 years. Current estimates peg the actual extinction rate over the last century to be up to 100 times higher than that. According to a comprehensive 2019 UN report, approximately 1 million of the globe’s 8 million species are at risk of extinction, with most major land habitats seeing the average abundance of native plant and animal life falling by 20% or more since 1900.
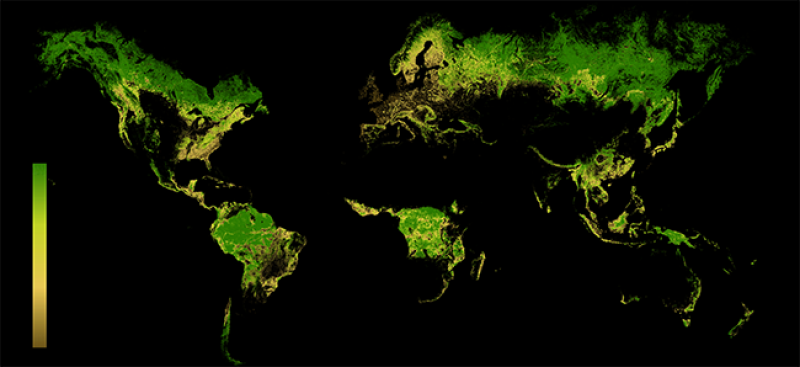
A 2019 forest landscape integrity index showing anthropogenic modification of remaining forest. Green represents most modification.
Scientific consensus agrees that throughout Earth’s history, the planet has undergone five major extinction events, the most recent (and most famous) of which occurred around 66 million years ago when an asteroid impact extinguished three-quarters of all plant and animal species, including the dinosaurs. The devastating biodiversity loss the world is witnessing today, scientists believe, is the sixth extinction.
There is, however, increasing awareness of our detrimental impacts on global biodiversity, and every day, more effort goes toward better understanding and protecting it. But scientists need data to give conservation endeavors a clearer picture of how species are faring, which wildlife management policies are succeeding and where to direct resources.
“We don’t have a baseline for a lot of these species,” Berger-Wolf said. “I’m not the one who makes the habitat assessments and improvements in the field. But what we can do is provide the data necessary to make those decisions, to understand what we’re losing, how fast we’re losing it and where actions would make the biggest difference.”
Walking Barcodes
It’s 2008 again. Berger-Wolf is with her collaborators in Kenya, and she is no longer skeptical. Her head, however, is spinning.
Berger-Wolf had asked a simple question: How did they collect the zebras’ information about their social networks? To her dismay, the answer was complicated.
Stripes are a zebra’s fingerprint — no two zebras have the same pattern. Matching individual zebras to their stripes allows researchers to keep tabs on them and determine their social networks and behaviors. To do that, they needed field assistants with good cameras and long lenses. Those field assistants had to take pictures, but they had to show the whole zebra. From just the right angle. With all its stripes. Once they got their perfect image, they’d upload it into a program and meticulously clicked on the animal’s outline, highlighting points to help the program identify what it was looking at. Then they did the same thing for all the zebra’s friends.
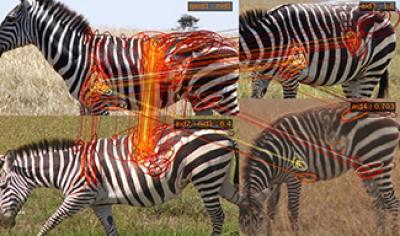
“Fifteen minutes later, I’m like, ‘OK this is insane. There has to be a better way.’” Berger-Wolf said. “And my colleagues were like, ‘Oh, Tanya, you always say there has to be a better way. Do you think you can do better?’ I’m like, ‘You wanna bet?’”
So Berger-Wolf got to work. For the next several years, she and her colleagues developed StripeSpotter, a program that detected and analyzed zebra outlines and stripes on its own. Instead of scientists combing through hundreds of photos and manually matching zebra stripes themselves, they could dump those images into the program, which would analyze the stripes for them — like scanning barcodes on items at the grocery store.
Walking barcodes,” Berger-Wolf said. “It caught people’s imagination. … And my colleague, Dan, he could actually use it in his work. It improved things. But it wasn’t great. I knew we could do better.”
A Species is Worth a Thousand Pictures
StripeSpotter generated a lot of buzz. If it could build out a zebra database, could something similar be created for other animals?
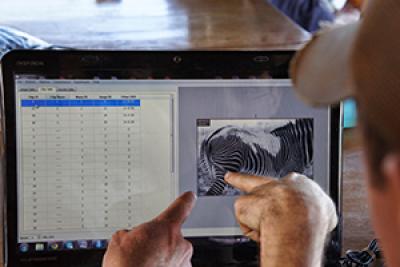
Berger-Wolf collaborated with Charles Stewart, a computer scientist at Rensselaer Polytechnic Institute, to find the answer, expanding StripeSpotter’s scope for a program that used a broader computer vision approach to identify any stripped, spotted or notched animal from photographs. After presenting early findings, requests came flooding in from ecologists from around the world asking if the program would work for species they were studying.
“We realized, ‘Wow, this is something that is useful and necessary and can really change how people in wildlife conservation and management can do their work,’” Berger-Wolf said.
In 2014, Berger-Wolf took leadership of the nonprofit Wild Me, an open-source platform for identifying and tracking wildlife. A year later, Wild Me launched its machine-learning software, the Image-Based Ecological Information System (IBEIS). It relied on images not just from researchers in the field, but from camera traps, drones, tourists and school field trips — anyone with a camera could contribute.
IBEIS was piloted during a two-day festival hosted by the Kenyan Wildlife Service. Students, animal enthusiasts and citizen scientists in Nairobi National Park took more than 10,000 photographs of zebras and giraffes, which were uploaded into the IBEIS system.
Wild Me's algorithms got to work, scouring through every pixel, identifying and categorizing the images into corresponding databases and counting how many of which animals there were in the park. By attributing time and location to each photo, scientists were able to paint a never-before-seen portrait that shed new light on zebra and giraffe population size, species interaction and movement patterns. This newfound information opened a whole new set of tools conservation experts could use to make more informed decisions and fine-tune their research.
While IBEIS pulled back the ecological curtain for scientists, it also empowered a new generation of Kenyan children to engage more fervently in their country’s wildlife conservation efforts. Taking photos in the field gave way to educational partnerships with antipoaching nonprofits, newly created wildlife clubs in schools nationwide and expanded field trips into nature preserves.
These ideas make connections,” Berger-Wolf said. “Connections not just between pixels in images, but also connections among people — from the 3-year-old nature lover to the 65-year-old zebra expert.”
Today, Wildbook, Wild Me’s flagship project, houses growing databases for not just zebras and giraffes, but for whale sharks, leopards, skunks, turtles, salamanders and other animals. It is used by nearly 900 wildlife researchers tracking over 188,000 individual animals, with photos pouring in from more than 15,000 citizens from around the world. Just recently, UNESCO named Wildbook one of its 100 AI projects worldwide that support the UN’s sustainable development goals.
“Machine learning and AI allow us not only to speed up the process, but to see the world from a different viewpoint,” Berger-Wolf said. “Our world is complex. Species are interrelated in complex ways. Looking at one animal or population or species at a time doesn’t tell the whole picture. Now, we have the technology to start asking different questions.”
In recognition of the International Day of Women and Girls in Science, Tanya Berger-Wolf recently conducted a Twitter takeover of the official National Science Foundation Twitter account.
A New Playing Field
In fall 2021, the National Science Foundation awarded Ohio State a $15 million grant to establish a new field of study and launch an interdisciplinary space dedicated to computation-enabled biological innovation and discovery.
The entity, the Imageomics Institute, is led by Berger-Wolf and housed at Ohio State's Translational Data Analytics Institue, of which Berger-Wolf is director, and will serve as the hub for scientific breakthroughs in the new field of imageomics. She recently discussed the new field in a presentation at the annual meeting of the American Associatation for the Advancement of Science.
Similar to genomics, which uses computation to study an organism’s genome, imageomics uses AI and machine learning to extract biological information from images of organisms. Imageomics and the Imageomics Institute, built on the foundations of Berger-Wolf’s early work, will allow scientists to pull the hidden insights from an otherwise unwieldly trove of images from museums, laboratories, other institutions and out in the field.
The data from these images won’t just give scientists an idea of population sizes and migration patterns. They’ll be able to, for example, determine if a baby zebra’s stripes are similar to its mothers and the underlying genetic mechanism behind it.
“This allows us to, for the first time ever, to start understanding in a different way the mechanisms of heritability of patterns, stripes and spots — to learn what makes one species look different from another, leading to a deeper understanding of foul billion years of evolution,” Berger-Wolf said. “Computers see the world differently than humans and can look at images and extract information that humans miss.”
From Kenya to StripeSpotter, IBEIS to Wild Me, Berger-Wolf’s imprint on the world of AI and wildlife conservation is as unique as the stripes across a zebra’s body or the notches etched into a whale shark’s fin. By stitching a clearer picture of global biodiversity, Berger-Wolf is establishing a more robust understanding of exactly what is at stake and a better idea of what path to take next.
This has been an incredible honor,” she said. “But it’s the outcome of a lot of people putting in the time, effort, passion and expertise. There are thousands throughout the world that make it happen, and our role in this is to enable them to do their jobs.”
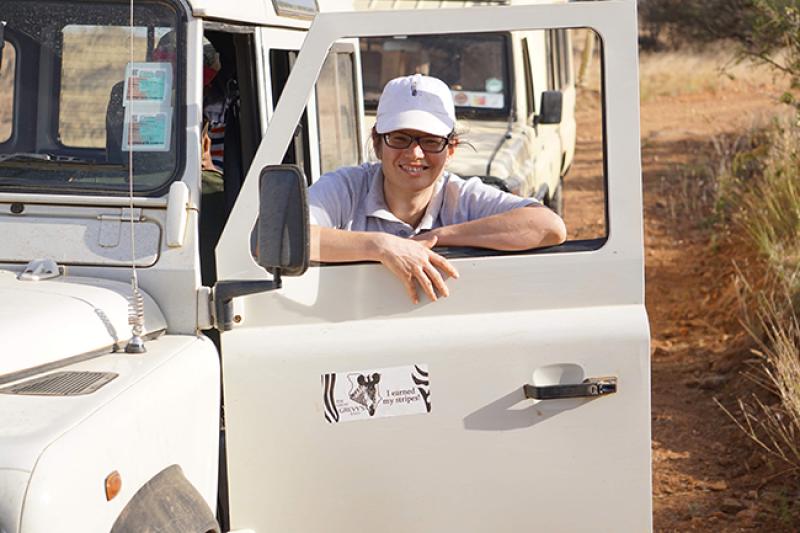